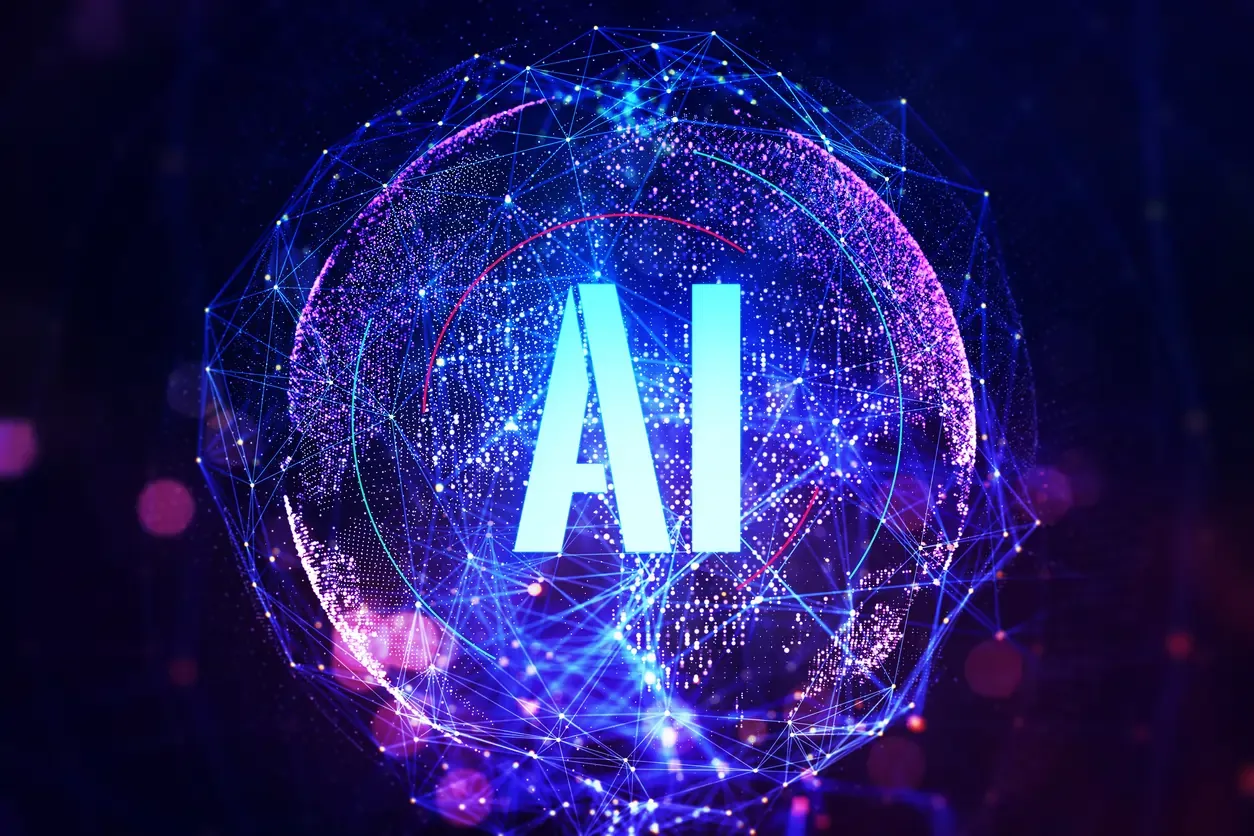
Financial audits are pivotal processes that ensure the accuracy, transparency, and integrity of financial statements. Traditionally, audits have been time-consuming, resource-intensive, and prone to human error. However, the advent of artificial intelligence (AI) and machine learning (ML) technologies has sparked a profound transformation in the landscape of financial audits. In this comprehensive blog post, we will delve into the multifaceted ways in which AI and ML are revolutionizing financial audits, exploring their applications, benefits, challenges, and future implications.
Understanding ai and machine learning in financial audits:
Before delving into the impact of AI and ML on financial audits, it’s essential to grasp the fundamental concepts of these technologies. AI involves the simulation of human intelligence in machines, enabling them to perform tasks that typically require human cognition, such as learning, reasoning, and problem-solving. ML, a subset of AI, focuses on the development of algorithms that allow computers to learn from and make predictions or decisions based on data.
Applications of ai and ml in financial audits:
Automated Data Analysis: AI and ML algorithms excel in analyzing vast volumes of financial data quickly and accurately, identifying patterns, anomalies, and trends that may indicate potential risks or errors. These technologies can significantly expedite the process of data analysis, allowing auditors to extract valuable insights more efficiently.
Risk Assessment: ML algorithms play a pivotal role in assessing financial risk by analyzing historical data, market trends, and other relevant factors. By leveraging predictive analytics, auditors can identify potential areas of risk and prioritize their audit procedures accordingly, thereby enhancing the effectiveness of the audit process.
Fraud Detection: AI-powered fraud detection systems employ sophisticated algorithms to detect unusual patterns or suspicious activities in financial transactions. These systems can analyze vast datasets in real-time, enabling auditors to identify and investigate potential instances of fraud more effectively.
Predictive Analytics: ML algorithms facilitate predictive analytics by forecasting future financial performance based on historical data. This capability enables auditors to anticipate potential issues or opportunities and make informed decisions to mitigate risks or capitalize on opportunities.
Continuous Monitoring: AI-enabled auditing tools enable continuous monitoring of financial transactions and processes in real-time. By leveraging real-time data analytics, auditors can detect anomalies or deviations from expected patterns promptly, allowing for timely intervention and risk mitigation.
Benefits of ai and ml in financial audits:
The integration of AI and ML technologies into financial audits offers a myriad of benefits, including:
Increased Efficiency: AI and ML technologies automate repetitive tasks, such as data entry, reconciliation, and pattern recognition, significantly reducing the time and resources required to conduct audits. By streamlining audit procedures, these technologies enable auditors to focus their efforts on more value-added activities, such as data analysis and decision-making.
Improved Accuracy: By leveraging advanced algorithms and data analytics techniques, AI and ML can identify errors, inconsistencies, and discrepancies with greater precision than traditional audit methods. This enhanced accuracy minimizes the risk of oversight or misinterpretation, ensuring the reliability and credibility of audit findings.
Enhanced Risk Management: AI-powered risk assessment tools enable auditors to identify potential risks and vulnerabilities more effectively, allowing organizations to implement proactive risk mitigation measures. By leveraging predictive analytics, auditors can anticipate emerging risks and take preemptive action to safeguard the organization’s financial integrity.
Cost Savings: The automation and efficiency improvements achieved through AI and ML technologies result in significant cost savings for organizations. By reducing the time and resources required to conduct audits, these technologies enable organizations to optimize their audit budgets and reallocate resources to other strategic initiatives.
Better Decision Making: AI and ML provide auditors with deeper insights and predictive capabilities, enabling them to make more informed decisions and recommendations to stakeholders. By leveraging data-driven insights, auditors can identify opportunities for process optimization, risk mitigation, and performance improvement, driving value for the organization.
Challenges and limitations:
Despite their numerous benefits, AI and ML technologies present several challenges and limitations in the context of financial audits, including:
Data Quality and Availability: AI and ML algorithms rely heavily on high-quality data for training and analysis. However, data quality issues, such as inaccuracies, incompleteness, and inconsistency, can undermine the effectiveness of these technologies. Ensuring data quality and availability remains a significant challenge for auditors and organizations.
Interpretability and Explainability: The black-box nature of some AI and ML algorithms can make it challenging for auditors to understand and interpret their decisions. This lack of interpretability raises concerns about accountability, transparency, and audit trail requirements. Auditors must ensure that AI and ML algorithms are transparent and explainable to stakeholders to maintain trust and credibility.
Regulatory Compliance: Regulatory requirements and standards governing financial audits may not always align with the use of AI and ML technologies. Ensuring compliance with regulatory requirements while leveraging AI and ML in financial audits poses a significant challenge for auditors and organizations. Regulatory bodies may need to develop specific guidelines and standards for the use of AI and ML in audits to address these compliance challenges effectively.
Ethical and Bias Concerns: AI and ML algorithms may exhibit bias or discrimination in their decision-making processes, leading to ethical concerns and potential legal implications. Auditors must ensure that AI and ML algorithms are trained on diverse and representative datasets to mitigate bias and discrimination effectively. Additionally, auditors must adhere to ethical guidelines and standards to ensure the responsible and ethical use of AI and ML technologies in financial audits.
Skill Gaps and Training Needs: Implementing AI and ML technologies in financial audits requires specialized skills and expertise. However, there is a significant skill gap in the auditing profession, with many auditors lacking the necessary knowledge and training in data analytics, machine learning, and AI ethics. Addressing these skill gaps and training needs is essential to ensure the successful adoption and integration of AI and ML technologies in financial audits.
Future implications and outlook:
The adoption of AI and ML technologies is expected to continue to grow in the field of financial audits, with significant implications for auditors, organizations, and regulators:
Evolution of Audit Practices: AI and ML will enable auditors to evolve their audit practices, moving towards more data-driven, proactive, and risk-focused approaches. By leveraging AI and ML technologies, auditors can enhance the efficiency, accuracy, and effectiveness of the audit process, driving value for organizations and stakeholders.
Collaboration and Partnership: Auditors may increasingly collaborate with technology vendors, data scientists, and other stakeholders to develop and deploy AI-powered auditing tools and solutions. By leveraging external expertise and resources, auditors can accelerate the adoption and integration of AI and ML technologies in financial audits, enhancing their capabilities and value proposition.
Regulatory Frameworks: Regulators are likely to develop and update regulatory frameworks to address the use of AI and ML in financial audits. These regulatory frameworks will aim to ensure compliance, transparency, and accountability in the use of AI and ML technologies, balancing innovation with regulatory requirements and standards.
Ethical Guidelines: The auditing profession may establish ethical guidelines and standards for the responsible and ethical use of AI and ML technologies in financial audits. These ethical guidelines will address concerns related to bias, privacy, fairness, and transparency, ensuring that AI and ML technologies are used in a manner that upholds ethical principles and values.
Skills Development: Auditors will need to acquire new skills and competencies in data analytics, machine learning, and AI ethics to effectively leverage these technologies in their practice. Training and upskilling initiatives will be essential to bridge the skill gap and ensure that auditors have the knowledge and expertise required to harness the full potential of AI and ML technologies in financial audits.
In conclusion, AI and machine learning are revolutionizing financial audits by enabling auditors to enhance efficiency, accuracy, and risk management capabilities. While these technologies offer significant benefits, they also pose challenges related to data quality, interpretability, regulatory compliance, ethics, and skills development. As the adoption of AI and ML continues to accelerate, auditors must navigate these challenges effectively and embrace opportunities for innovation and transformation in the auditing profession. By leveraging AI and ML technologies, auditors can optimize audit processes, mitigate risks, and drive value for organizations and stakeholders, paving the way for a more robust and resilient audit ecosystem in the digital age.