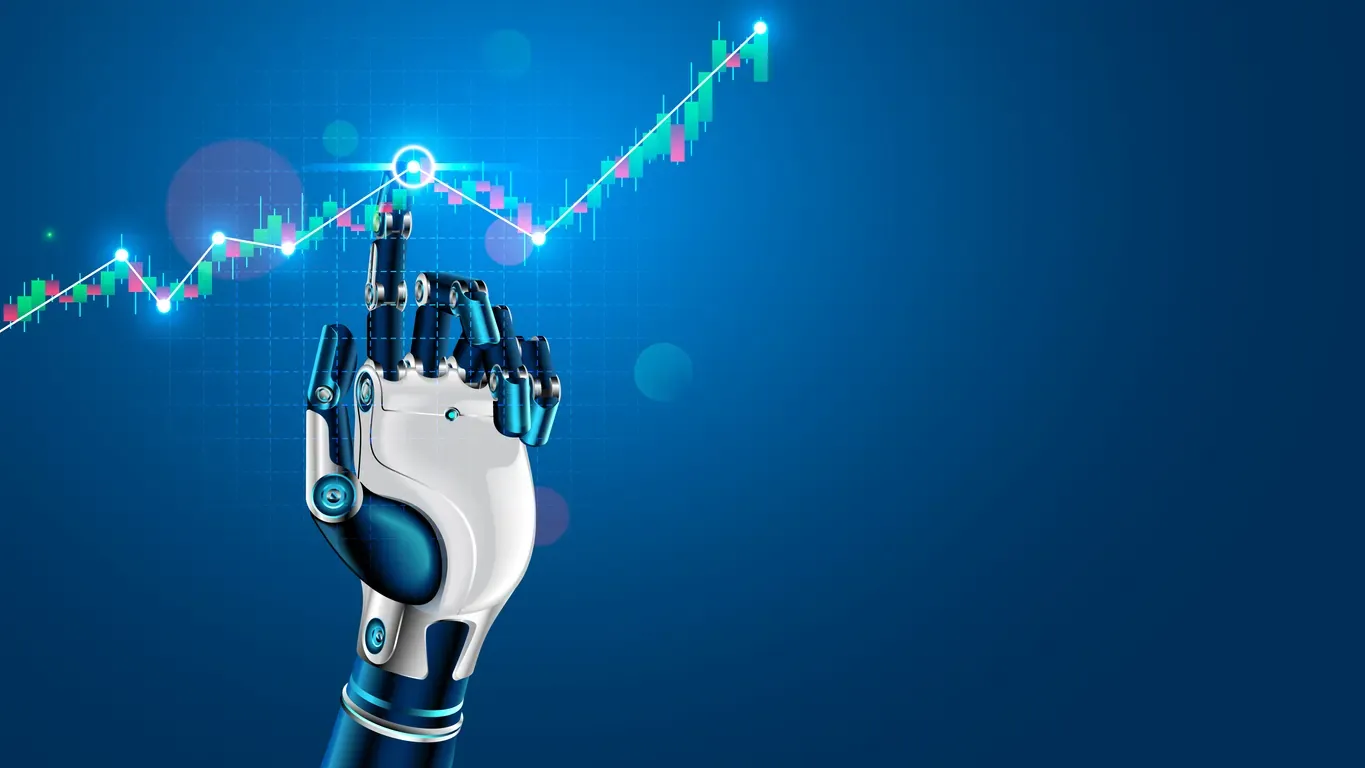
The Impact of Machine Learning on Enhancing Credit Decision Models
Introduction
Machine learning is revolutionizing the way financial institutions make credit decisions, offering more accurate and efficient models than ever before. This article explores the impact of machine learning on enhancing credit decision models, including key trends, benefits, challenges, and future prospects.
- Machine Learning in Credit Decision Models
Machine learning is a subset of artificial intelligence that enables computers to learn from data and make decisions without being explicitly programmed. In the context of credit decision models, machine learning algorithms analyze large datasets to identify patterns and predict creditworthiness more accurately than traditional models.
- Key Trends in Machine Learning for Credit Decision Models
- a) Predictive Analytics: Machine learning algorithms can analyze historical data to predict future credit risk more accurately, enabling lenders to make more informed decisions.
- b) Automated Decision Making: Machine learning can automate the credit decision process, reducing the time and resources required to assess loan applications.
- Benefits of Machine Learning in Credit Decision Models
- a) Improved Accuracy: Machine learning algorithms can analyze vast amounts of data to identify subtle patterns and trends that traditional models may miss, leading to more accurate credit decisions.
- b) Faster Decisions: Machine learning can automate the credit decision process, reducing the time it takes to approve or deny a loan application.
- Challenges of Implementing Machine Learning in Credit Decision Models
- a) Data Quality: Machine learning algorithms require high-quality data to make accurate predictions, which can be challenging to obtain in practice.
- b) Interpretability: Some machine learning algorithms, such as deep learning models, are complex and difficult to interpret, making it challenging for lenders to understand how decisions are made.
- Future Prospects of Machine Learning in Credit Decision Models
- a) Continued Advancements: As machine learning technologies continue to evolve, they are likely to become even more accurate and efficient in predicting credit risk.
- b) Regulatory Compliance: Regulatory agencies are increasingly focused on ensuring that machine learning models used in credit decision-making are fair and transparent, which will drive further innovation in the field.
- Best Practices for Implementing Machine Learning in Credit Decision Models
- a) Data Quality: Ensuring that the data used to train machine learning models is accurate, up-to-date, and representative of the population being assessed.
- b) Model Interpretability: Using machine learning models that are interpretable and can provide insights into how decisions are made.
- Case Studies: Examples of Successful Implementation of Machine Learning in Credit Decision Models
- a) ZestFinance: ZestFinance uses machine learning to analyze alternative data sources and provide credit scores for individuals with limited credit histories, enabling lenders to make more inclusive lending decisions.
- b) Upstart: Upstart uses machine learning to assess the creditworthiness of borrowers based on factors such as education and employment history, providing more accurate risk assessments than traditional credit scores.
Conclusion
In conclusion, machine learning is transforming credit decision models by enabling lenders to make more accurate and efficient decisions. As machine learning technologies continue to evolve, they are likely to play an increasingly important role in the financial industry, leading to more inclusive and efficient lending practices.